Matplotloom 0.8.1¶
Matplotloom is a Python library for creating animations from matplotlib figures. It provides a simple interface to save individual frames and compile them into animations (video or GIF) using ffmpeg.
Why use matplotloom?¶
To visualize simulation output for computational fluid dynamics I’ve had to make long animations with complex figures for a long time. The animations consist of thousands of frames and the figures are too complex for FuncAnimation
and ArtistAnimation
. This package aims to simplify and massively speed up the process of making these kinds of animations.
The main idea behind matplotloom is to describe how to generate each frame of your animation from scratch, instead of generating an animation by modifying one existing plot. This simplifies generating animations. See the examples below and how the code inside the
for
loops is plain and familiar matplotlib. It also ensures that every feature can be animated and that the generation process can be easily parallelized.matplotlib has two tools for making animations:
FuncAnimation
andArtistAnimation
. But to use them you have to write your plotting code differently to modify an existing frame. This makes it difficult to go from plotting still figures to making animations. And some features are non-trivial to animate.celluloid is a nice package for making matplotlib animations easily, but as it relies on
ArtistAnimation
under the hood it does come with some limitations such as not being able to animate titles. It also hasn’t been maintained since 2018.Plotting many frames (hundreds to thousands+) can be slow but with matplotloom you can use a parallel
Loom
to plot each frame in parallel, speeding up the animation process significantly especially if you can dedicate many cores to plotting.
Some notes to users¶
You can use
loom.show()
to display animations in Jupyter notebooks.Anxious about animation progress? Pass
verbose=True
or use tqdm to monitor progress.Animations taking too long to make or do you have tons of frames? You can parallelize frame creating by looming in parallel.
You have to call
loom.save_frame(fig)
for each frame (see the examples). While theLoom
object can be made to do this automatically it would have to create and own theFigure
instance and I wanted full control over the creation of theFigure
for maximum flexibility.matplotloom is going to be slow. But it’s flexible and compatible with all of matplotlib! The real speedup comes from parallelizing frame creation, especially if you have a ton of frames to make.
Installation¶
matplotloom is published on PyPI so you can install matplotloom via pip
pip install matplotloom
or uv
uv add matplotloom
or poetry
poetry add matplotloom
or conda
conda install matplotloom
matplotloom requires Python 3.10+ and is continuously tested on Linux, Windows, and Mac. Ensure you have ffmpeg
installed so that animations can be generated.
Examples¶
Sine wave¶
import numpy as np
import matplotlib.pyplot as plt
from matplotloom import Loom
with Loom("sine_wave_animation.gif", fps=30) as loom:
for phase in np.linspace(0, 2*np.pi, 100):
fig, ax = plt.subplots()
x = np.linspace(0, 2*np.pi, 200)
y = np.sin(x + phase)
ax.plot(x, y)
ax.set_xlim(0, 2*np.pi)
loom.save_frame(fig)
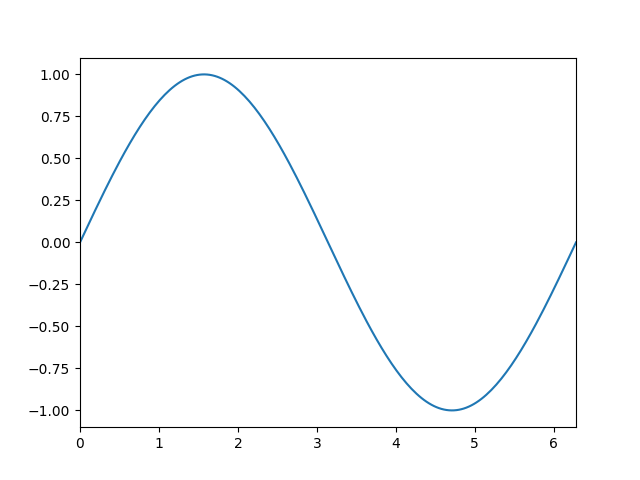
Rotating circular sine wave¶
import numpy as np
import matplotlib.pyplot as plt
from matplotloom import Loom
with Loom("rotating_circular_sine_wave.mp4", fps=10) as loom:
for i in range(36):
fig, ax = plt.subplots(figsize=(12, 8), subplot_kw={"projection": "3d"})
X = np.arange(-5, 5, 0.25)
Y = np.arange(-5, 5, 0.25)
X, Y = np.meshgrid(X, Y)
R = np.sqrt(X**2 + Y**2)
Z = np.sin(R)
surf = ax.plot_surface(X, Y, Z, cmap="coolwarm")
ax.view_init(azim=i*10)
ax.set_zlim(-1.01, 1.01)
fig.colorbar(surf, shrink=0.5, aspect=5)
loom.save_frame(fig)
Bessel wave¶
Compare with animatplot’s blocks example. With matplotloom you just use regular matplotlib abstractions.
import numpy as np
import matplotlib.pyplot as plt
from scipy.special import j0
from cmocean import cm
from matplotloom import Loom
def bessel_wave(r, t, k, omega, A):
return A * j0(k*r - omega*t)
def create_frame(x, y, t):
fig, (ax1, ax2) = plt.subplots(1, 2, figsize=(12, 5))
r = np.sqrt(x**2 + y**2)
z = bessel_wave(r, t, k=2, omega=1, A=1)
pcm = ax1.pcolormesh(x, y, z, cmap=cm.balance, shading='auto', vmin=-1, vmax=1)
fig.colorbar(pcm, ax=ax1)
ax1.set_title(f"Bessel wave: t = {t:.3f}")
ax1.set_xlabel("x")
ax1.set_ylabel("y")
ax1.set_xlim(-10, 10)
ax1.set_ylim(-10, 10)
ax1.set_aspect("equal", adjustable="box")
mid = z.shape[0] // 2
ax2.plot(x[mid], z[mid])
ax2.set_xlim(x.min(), x.max())
ax2.set_ylim(-1.1, 1.1)
ax2.set_title("Cross-section at y = 0")
ax2.set_xlabel("x")
ax2.set_ylabel("z")
return fig
loom = Loom(
"bessel_wave.mp4",
fps = 30,
overwrite = True,
verbose = True,
savefig_kwargs = {
"dpi": 100,
"bbox_inches": "tight"
}
)
with loom:
x = np.linspace(-10, 10, 500)
y = np.linspace(-10, 10, 500)
x, y = np.meshgrid(x, y)
for t in np.linspace(0, 50, 300):
fig = create_frame(x, y, t)
loom.save_frame(fig)
Double pendulum¶
Compare with matplotlib’s double pendulum example.
import numpy as np
import matplotlib.pyplot as plt
from scipy.integrate import solve_ivp
from tqdm import tqdm
from matplotloom import Loom
g = 9.80665 # standard acceleration of gravity [m/s²]
l1, l2 = 1, 1 # pendulum arms lengths [m]
m1, m2 = 1, 1 # pendulum masses [kg]
# Calculate dy/dt where y = [θ1, ω1, θ2, ω2].
def derivatives(t, state):
θ1, ω1, θ2, ω2 = state
dydt = np.zeros_like(state)
dydt[0] = ω1
dydt[2] = ω2
Δθ = θ2 - θ1
denominator1 = (m1 + m2) * l1 - m2 * l1 * np.cos(Δθ)**2
dydt[1] = (m2 * l1 * ω1**2 * np.sin(Δθ) * np.cos(Δθ)
+ m2 * g * np.sin(θ2) * np.cos(Δθ)
+ m2 * l2 * ω2**2 * np.sin(Δθ)
- (m1 + m2) * g * np.sin(θ1)) / denominator1
denominator2 = (l2 / l1) * denominator1
dydt[3] = (-m2 * l2 * ω2**2 * np.sin(Δθ) * np.cos(Δθ)
+ (m1 + m2) * g * np.sin(θ1) * np.cos(Δθ)
- (m1 + m2) * l1 * ω1**2 * np.sin(Δθ)
- (m1 + m2) * g * np.sin(θ2)) / denominator2
return dydt
t_span = (0, 20)
y0 = [np.pi/2, 0, np.pi/2, 0]
sol = solve_ivp(derivatives, t_span, y0, dense_output=True)
times = np.linspace(t_span[0], t_span[1], 1000)
θ1, ω1, θ2, ω2 = sol.sol(times)
x1 = l1 * np.sin(θ1)
y1 = -l1 * np.cos(θ1)
x2 = x1 + l2 * np.sin(θ2)
y2 = y1 - l2 * np.cos(θ2)
loom = Loom(
"double_pendulum.mp4",
fps = 60,
overwrite = True,
savefig_kwargs = {"bbox_inches": "tight"}
)
with loom:
for i, t in tqdm(enumerate(times), total=len(times)):
fig, ax = plt.subplots(figsize=(8, 8))
ax.plot(
[0, x1[i], x2[i]],
[0, y1[i], y2[i]],
linestyle = "solid",
marker = "o",
color = "black",
linewidth = 3
)
ax.plot(
x2[:i+1],
y2[:i+1],
linestyle = "solid",
linewidth = 2,
color = "red",
alpha = 0.5
)
ax.set_title(f"Double Pendulum: t = {t:.3f}s")
ax.set_xlim(-2.2, 2.2)
ax.set_ylim(-2.2, 2.2)
ax.set_aspect("equal", adjustable="box")
loom.save_frame(fig)
Night time shading¶
matplotloom works out of the box with anything that is built on top of matplotlib. Here we’re extending a Cartopy example.
import datetime
import matplotlib.pyplot as plt
import cartopy.crs as ccrs
from cartopy.feature.nightshade import Nightshade
from joblib import Parallel, delayed
from matplotloom import Loom
def plot_frame(day_of_year, loom, frame_number):
date = datetime.datetime(2024, 1, 1, 12) + datetime.timedelta(days=day_of_year-1)
fig = plt.figure(figsize=(15, 5))
proj1 = ccrs.Orthographic(central_longitude=0, central_latitude=30)
proj2 = ccrs.Orthographic(central_longitude=120, central_latitude=0)
proj3 = ccrs.Orthographic(central_longitude=240, central_latitude=-30)
ax1 = fig.add_subplot(1, 3, 1, projection=proj1)
ax2 = fig.add_subplot(1, 3, 2, projection=proj2)
ax3 = fig.add_subplot(1, 3, 3, projection=proj3)
fig.suptitle(f"Night time shading for {date} UTC")
ax1.stock_img()
ax1.add_feature(Nightshade(date, alpha=0.2))
ax2.stock_img()
ax2.add_feature(Nightshade(date, alpha=0.2))
ax3.stock_img()
ax3.add_feature(Nightshade(date, alpha=0.2))
loom.save_frame(fig, frame_number)
loom = Loom(
"night_time_shading.mp4",
fps = 10,
overwrite = True,
parallel = True,
verbose = True,
savefig_kwargs = {
"bbox_inches": "tight"
}
)
with loom:
n_days_2024 = 366
days_of_year = range(1, n_days_2024 + 1)
Parallel(n_jobs=-1)(
delayed(plot_frame)(day_of_year, loom, i)
for i, day_of_year in enumerate(days_of_year)
)
Looming in parallel¶
By passing parallel=True
when creating a Loom
, you can save frames using loom.save_frame(fig, frame_number)
which allows you to plot and save all your frames in parallel. One easy way to leverage this is by using joblib to parallelize the for loop. For example, here’s how you can parallelize the simple sine wave example:
import numpy as np
import matplotlib.pyplot as plt
from matplotloom import Loom
from joblib import Parallel, delayed
def plot_frame(phase, frame_number, loom):
fig, ax = plt.subplots()
x = np.linspace(0, 2*np.pi, 200)
y = np.sin(x + phase)
ax.plot(x, y)
ax.set_xlim(0, 2*np.pi)
loom.save_frame(fig, frame_number)
with Loom("parallel_sine_wave.gif", fps=30, parallel=True) as loom:
phases = np.linspace(0, 2*np.pi, 100)
Parallel(n_jobs=-1)(
delayed(plot_frame)(phase, i, loom)
for i, phase in enumerate(phases)
)
Reference¶
- class matplotloom.Loom(output_filepath: Path | str, frames_directory: Path | str | None = None, fps: int = 30, keep_frames: bool = False, overwrite: bool = False, verbose: bool = False, parallel: bool = False, show_ffmpeg_output: bool = False, savefig_kwargs: Dict[str, Any] | None = None)¶
A class for creating animations from matplotlib figures.
This class provides functionality to save individual frames and compile them into an animation (video or GIF) using ffmpeg.
- Parameters:
output_filepath (Union[Path, str]) – Path to save the final animation file.
frames_directory (Union[Path, str, None], optional) – Directory to save individual frames. If None, a temporary directory is used.
fps (int, optional) – Frames per second for the output animation. Default is 30.
keep_frames (bool, optional) – Whether to keep individual frame files after creating the animation. Default is False.
overwrite (bool, optional) – Whether to overwrite the output file if it already exists. Default is False.
verbose (bool, optional) – Whether to print detailed information during the process. Default is False.
parallel (bool, optional) –
Whether to enable parallel frame saving. Default is False. When True, this enables a mode where frames can be saved concurrently, significantly speeding up the animation creation process for computationally intensive plots or large numbers of frames.
- In parallel mode:
The save_frame method requires an explicit frame number.
Frames can be created and saved in any order.
The user is responsible for parallelizing the frame creation process, typically using tools like joblib, multiprocessing, or concurrent.futures.
show_ffmpeg_output (bool, optional) – Whether to show ffmpeg output when saving the video. Default is False. When True, the ffmpeg command and its stdout/stderr output will be printed during video creation, regardless of the verbose setting.
savefig_kwargs (dict, optional) – Additional keyword arguments to pass to matplotlib’s savefig function. Default is {}.
- Raises:
FileExistsError – If the output file already exists and overwrite is False.
- save_frame(fig: Figure, frame_number: int | None = None) None ¶
Save a single frame of the animation.
- Parameters:
fig (matplotlib.figure.Figure) – The matplotlib figure to save.
frame_number (int, optional) – The frame number (required if parallel=True).
- save_video() None ¶
Compile saved frames into a video or GIF using ffmpeg.
This method uses ffmpeg to create the final animation from the saved frames. The output format is determined by the file extension of the output filepath.
- show(**kwargs) Video | Image ¶
Display the created animation in a Jupyter notebook.
This method returns an IPython display object that can be used to show the animation directly in a Jupyter notebook cell. The type of object returned depends on the file format of the animation.
- Parameters:
**kwargs – Keyword arguments that will be passed to either IPython.display.Video or IPython.display.Image constructor depending on the file format.
- Returns:
An IPython Video object for MP4 or MKV formats, or an IPython Image object for GIF or APNG formats. These objects can be displayed directly in a Jupyter notebook.
- Return type:
Union[IPython.display.Video, IPython.display.Image]
Notes
This method is designed to work in Jupyter notebooks. It may not have the desired effect in other Python environments.
The animation file must have been successfully created by the save_video method before calling this method.